AI Diaries: Weekly Updates #8
- Esra Kuzu
- Sep 15, 2024
- 7 min read
Updated: Sep 21, 2024
Welcome to this month's edition of AI Diaries: Weekly Updates! This week’s AI Diaries highlights some latest AI advancements and innovations.
Google has introduced HeAR, an innovative AI model that uses smartphone microphones to detect diseases by analyzing sounds like coughs and breathing patterns, aiming to provide early-stage disease detection in regions with limited healthcare resources. AlphaProteo is making waves by designing novel protein binders for biological research, offering improved success rates and binding strengths that could accelerate drug discovery and disease prevention. OpenAI has unveiled its new model, o1, which excels in complex problem-solving tasks such as coding and mathematics through advanced reasoning capabilities. Microsoft’s TorchGeo 0.6.0 toolkit simplifies the integration of geospatial data into machine learning workflows, enhancing research in environmental monitoring and urban planning. FutureHouse Inc.'s PaperQA2 is revolutionizing scientific literature reviews by autonomously retrieving, summarizing, and detecting contradictions in research papers, dramatically speeding up the review process for scientists. Finally, Ashvini Kumar Jindal and his team have developed Llama-3.1-Storm-8B, an advanced AI model with 8 billion parameters that excels in natural language processing tasks and promises to transform industries such as customer service, content creation, and healthcare, though it also raises important ethical concerns.
These stories offer valuable insights and showcase the remarkable progress being made in technology and AI. Enjoy the read, and we invite you to share your thoughts in the comments below!
Let’s dive in.
Google's AI Breakthrough: Diagnosing Diseases Through Smartphone Sounds
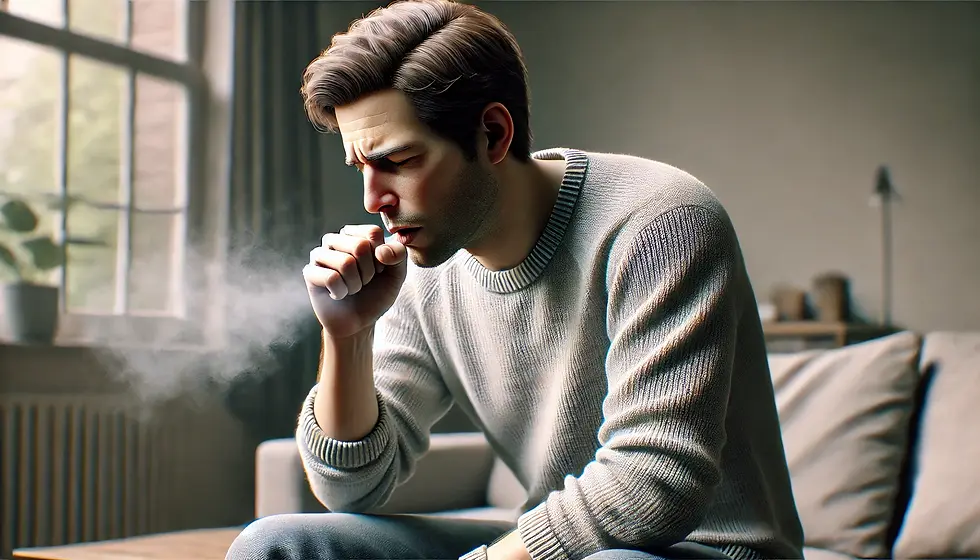
TL;DR: Google trained an AI model called HeAR on 300 million audio samples, including coughs and breathing sounds, to detect diseases like tuberculosis through smartphone microphones. This technology aims to provide accessible, early-stage disease detection in regions with limited healthcare access, particularly for respiratory illnesses.
What's The Essence?: Google's AI uses sound signals to detect early symptoms of diseases such as tuberculosis. By analyzing subtle differences in coughs and breathing patterns, the model provides a non-invasive, mobile solution for diagnosing and monitoring respiratory health, especially in underserved areas.
How Does It Tick?:The AI model was trained on a large dataset of publicly available audio samples, including medical screenings and online videos. It uses pattern recognition to identify signs of disease in human sounds. Integrated into smartphones, this technology offers an easy-to-deploy tool that analyzes audio data and provides diagnostic insights without needing traditional medical devices.
Why It Matters?: This technology could revolutionize healthcare by making disease detection accessible in remote and underserved areas. Tuberculosis, which causes millions of deaths annually, often goes undiagnosed due to the lack of diagnostic tools. With AI-based audio analysis on smartphones, early detection becomes possible, enabling timely treatment and potentially saving thousands of lives.
---
AlphaProteo: AI-Designed Proteins Revolutionize Drug Discovery
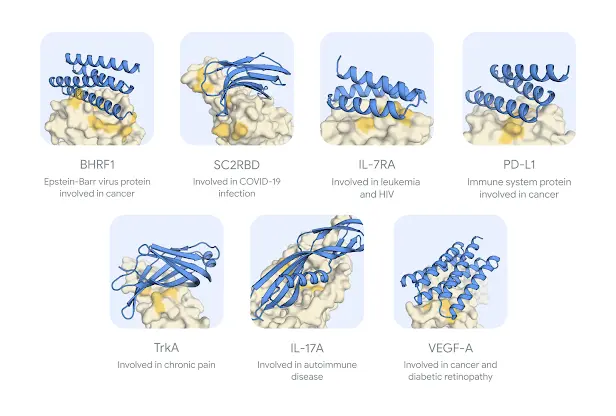
TL;DR: AlphaProteo is an AI system that designs novel protein binders for biological research, achieving higher success rates and binding strengths than existing methods. It generates proteins that bind to targets like the SARS-CoV-2 spike protein and cancer-related proteins, with potential applications in drug discovery and disease prevention.
What's The Essence?: AlphaProteo creates new, high-affinity protein binders for specific biological targets, advancing beyond protein structure prediction tools like AlphaFold. It accelerates the design process for proteins that could be used in drug development, disease research, and more, with better experimental outcomes than current methods.
How Does It Tick?: AlphaProteo is powered by machine learning models trained on large datasets of protein structures. By analyzing how molecules bind, it generates protein designs that are experimentally tested for effectiveness. Its AI-driven approach significantly reduces the time and labor required to develop useful protein binders by achieving higher binding success rates and affinities.
Why It Matters?: This AI breakthrough can revolutionize fields like drug discovery, diagnostics, and biotechnology. It enables faster and more efficient design of proteins that are essential in treating diseases, preventing viral infections, and tackling biological challenges, potentially saving time in medical research and therapeutic development.
---
OpenAI's o1 Model: A New Era of Complex Problem Solving in AI
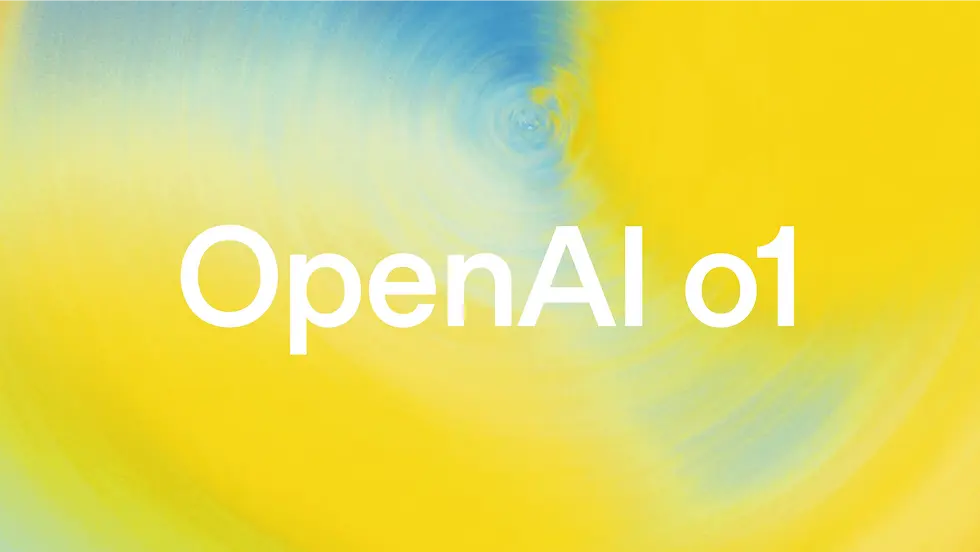
TL;DR: OpenAI has released o1, a new model with advanced reasoning capabilities, designed to solve complex problems like coding and mathematics better than previous models. It uses reinforcement learning and a "chain of thought" process to break down problems step-by-step. While more accurate, it is slower and more expensive than GPT-4o. This model is a step towards human-like intelligence but still lacks some capabilities like browsing and image processing.
What's The Essence?: The o1 model is OpenAI's first major step toward improving reasoning in AI, allowing it to handle more complex queries and solve multi-step problems, particularly in coding and math. It represents a shift from pattern-matching to more human-like problem-solving, although it's still early in development.
How Does It Tick?: o1 works by employing a new training method based on reinforcement learning, which rewards or penalizes the model as it solves tasks. This, combined with a "chain of thought" process, allows it to approach problems step-by-step, mimicking human-like reasoning. This methodology makes it better at complex tasks, even though it's slower and more expensive to run compared to its predecessors.
Why It Matters?: The o1 model pushes AI closer to human-level reasoning, making it better suited for applications in fields like coding, mathematics, and potentially science and medicine. By improving AI's ability to reason, o1 could unlock significant breakthroughs in autonomous systems and complex decision-making processes. However, its high cost and slower performance mean it's still an experimental leap toward a more advanced AI future.
For More: https://openai.com/o1/
---
TorchGeo 0.6.0: Simplifying Geospatial Data for AI Research
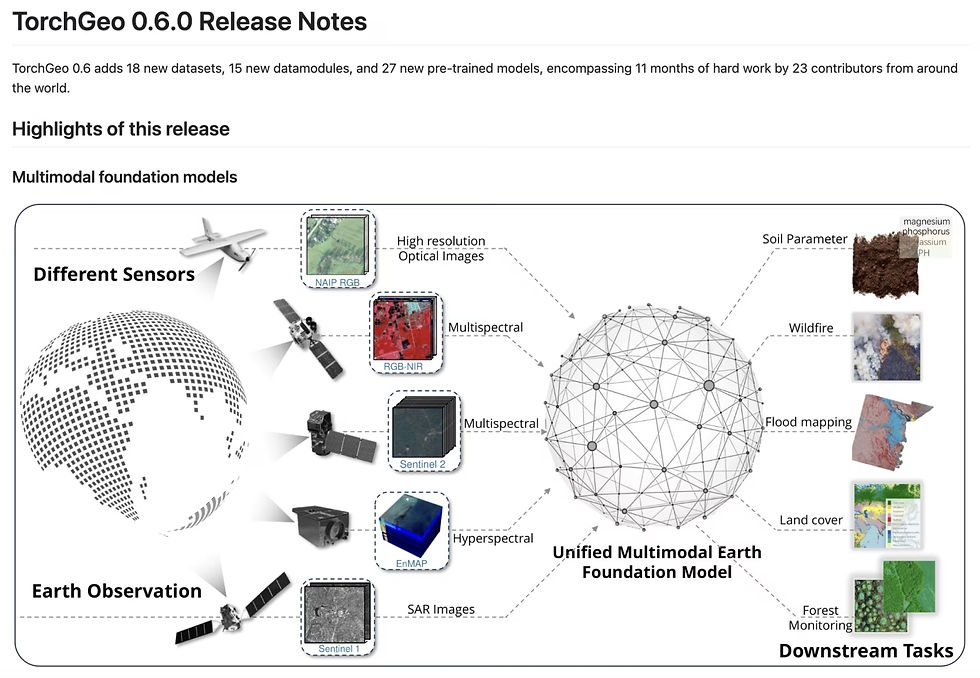
TL;DR: TorchGeo 0.6.0, released by Microsoft, is a modular toolkit designed to simplify working with geospatial data in machine learning workflows. It provides curated datasets, sampling strategies, specialized data transforms, and pre-trained models, all aimed at reducing the complexity and computational demands of integrating remote sensing data. With support for distributed training via PyTorch Lightning, it accelerates research in areas like environmental monitoring and urban planning.
What's The Essence?: The essence of TorchGeo 0.6.0 is its ability to streamline the integration of complex, heterogeneous geospatial data into machine learning workflows. It solves longstanding challenges by offering a standardized, open-source toolkit that combines datasets, preprocessing tools, and pre-trained models tailored specifically for remote sensing and geospatial applications.
How Does It Tick?
Standardized datasets (e.g., Sentinel-2, PlanetScope) and easy API access.
Automated preprocessing tools, such as data augmentation and specialized transforms for geospatial data (e.g., cloud masking).
Flexible sampling techniques like random, grid, and stratified sampling to create balanced datasets.
Pre-trained models for tasks like semantic segmentation and classification, optimized for fine-tuning on geospatial data.
Distributed training support through PyTorch Lightning, allowing scalable model training across multiple GPUs or machines.
Why It Matters?: TorchGeo 0.6.0 is important because it tackles the barriers that have long hindered progress in geospatial machine learning, such as data heterogeneity, complexity, and high computational costs. By offering a comprehensive, easy-to-use toolkit, it empowers researchers and developers to work more efficiently with geospatial data. This innovation has broad implications, from enhancing environmental monitoring to improving urban planning and disaster response, accelerating the development of data-driven solutions in critical areas.
-
PaperQA2: AI-Powered Literature Reviews Redefine Scientific Research
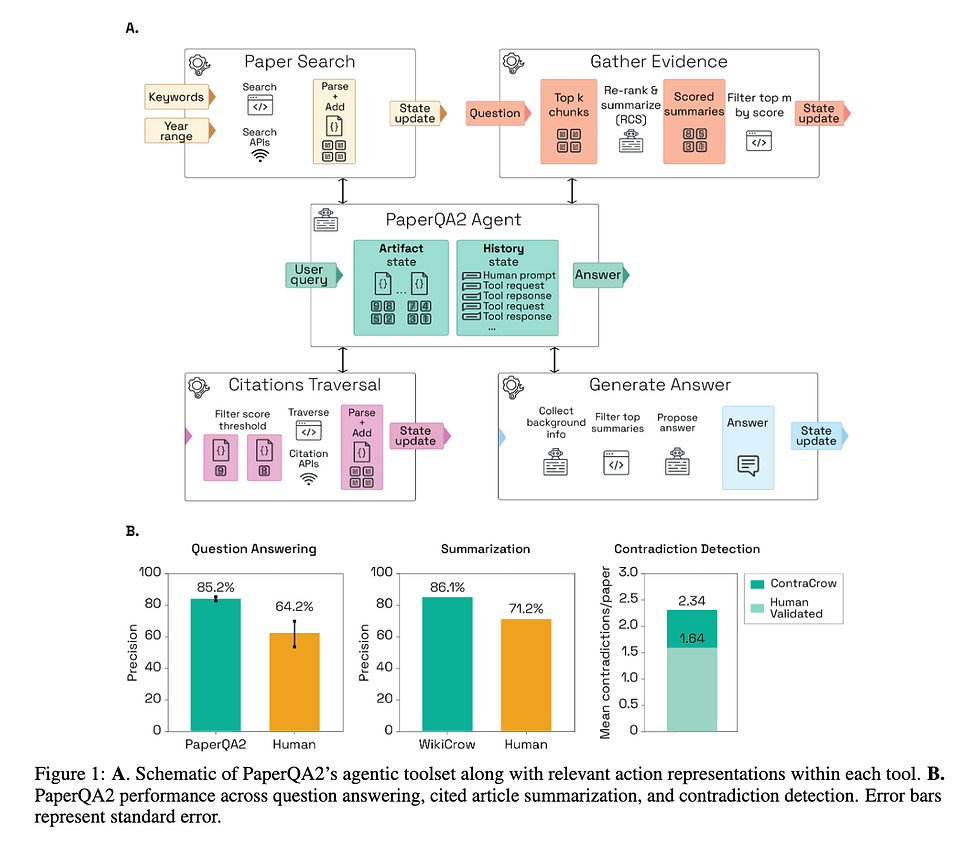
TL;DR: PaperQA2, developed by FutureHouse Inc., is the first AI agent capable of autonomously conducting scientific literature reviews. It efficiently retrieves, summarizes, and detects contradictions in scientific papers, surpassing existing AI tools in accuracy and precision, and even outperforming human experts in certain tasks. This AI system dramatically speeds up the research process, making it easier for scientists to manage vast volumes of literature.
What's The Essence?: PaperQA2 automates the most tedious and complex parts of scientific literature reviews, like searching for relevant studies, summarizing intricate topics, and identifying contradictions. It provides highly accurate and detailed responses, empowering researchers to navigate and synthesize large bodies of scientific knowledge with unprecedented efficiency.
How Does It Tick?
Paper Search: Finds relevant scientific papers using keyword searches.
Grobid Parsing: Breaks papers into machine-readable chunks.
Relevance Ranking: Ranks chunks using the "Gather Evidence" tool.
Reranking and Summarization: Refines retrieved information with RCS to generate precise summaries.
Citation Traversal: Tracks relevant sources to improve literature coverage.
Why It Matters?: PaperQA2 revolutionizes scientific research by saving time and increasing accuracy in literature reviews, a process that can often overwhelm human researchers. With its ability to process and analyze vast amounts of data faster and more reliably than human experts, PaperQA2 enables researchers to focus on innovation and discovery, accelerating progress in various scientific fields.
-
Llama-3.1-Storm-8B: The AI Model Transforming Natural Language Processing
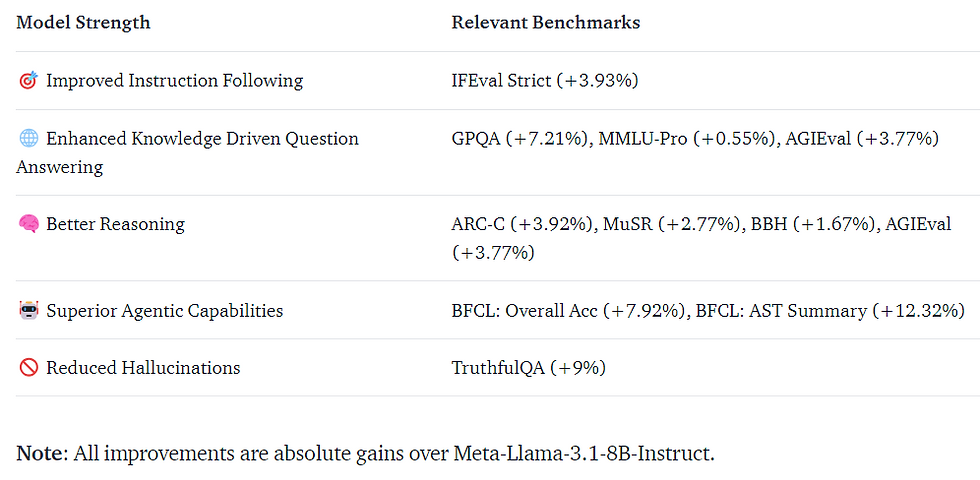
TL;DR: Llama-3.1-Storm-8B, developed by Ashvini Kumar Jindal and his team, is a groundbreaking AI model with 8 billion parameters that outperforms Meta AI’s Llama-3.1-8B-Instruct and Hermes-3-Llama-3.1-8B models across various benchmarks. It excels in natural language processing (NLP) tasks, offering improved instruction-following, question answering, and reduced hallucination rates. With its real-time, context-aware capabilities, it’s poised to transform industries like customer service, content creation, translation, and healthcare, while also raising ethical concerns.
What's The Essence?: Llama-3.1-Storm-8B is a highly advanced language model designed to outperform existing models in natural language understanding and generation. With its 8 billion parameters, it delivers superior accuracy, context awareness, and real-time performance, making it valuable for applications such as automated customer support, content generation, and healthcare diagnostics.
How Does It Tick?: The model works by leveraging a transformer-based architecture and advanced training techniques, including targeted fine-tuning and self-curation. It selects high-quality examples from vast datasets to enhance its performance in specific tasks like instruction following, question answering, and function calling. With optimizations for real-time processing, the model delivers quick, contextually accurate, and grammatically coherent responses. It balances computational efficiency with output quality, making it ideal for industries requiring fast, high-quality text generation.
Why It Matters?: Llama-3.1-Storm-8B sets a new standard in NLP, offering superior performance across various tasks compared to competitors. It enables businesses and industries to automate complex language-based tasks more effectively, saving time and resources. However, the model’s power also poses ethical risks, such as potential misuse for creating convincing fake content or amplifying biases, necessitating responsible deployment and ongoing improvements to address these challenges.
If you've read this far, you're amazing! 🌟 Keep striving for knowledge and continue learning! 📚✨
Comments